Digital Twins
Digital twins (DTs) are surrogates or digital replicas of physical assets or processes. These digital replicas allow companies to simulate and analyze a multitude of scenarios before making changes or implementing them in the real world. The correct utilization of digital twins gives companies a competitive advantage.
Improved Design: DTs allow engineers to test and optimize designs before the physical assets are built. This results in cost savings as well as improvement in the over product quality.
Increased Efficiency: By simulating different scenarios and optimizing processes DTs can help companies identify ways to improve efficiency and reduce waste.
Predictive Maintenance: DTs can be used to monitor physical assets and forecast when maintenance is required, reducing downtime and maintenance costs will increasing asset life.
Real-time Decision Making: DTs provide real-time data and insights that can help companies make faster and more informed decisions.
Enhanced Safety: DTs can simulate extreme events, dangerous scenarios and test safety protocols allowing companies to identify and mitigate safety risks.
Remote Monitoring: DTs can be used to monitor assets and processes remotely, reducing the need for on-site personnel.
The Difference with AI-Powered Digital Twins
Reduced Order Models (ROMs): Non-AI (classical) approach
There are two forms of ROMs, model-based or data-driven. Model-based ROMs rely on mathematical and physical understanding of the underlying physics that guide a process. Data-driven methods use input-output, high fidelity data to build the ROM.
Deep Operator Network (DeepONet): AI based approach
DeepONet utilizes the recent advancements in deep learning to build fast surrogates. In particular DeepONet demonstrates the ability of neural networks to approximate mathematical operators. Recent developments in physics-informed learning has enabled the incorporation of both data and physicals models
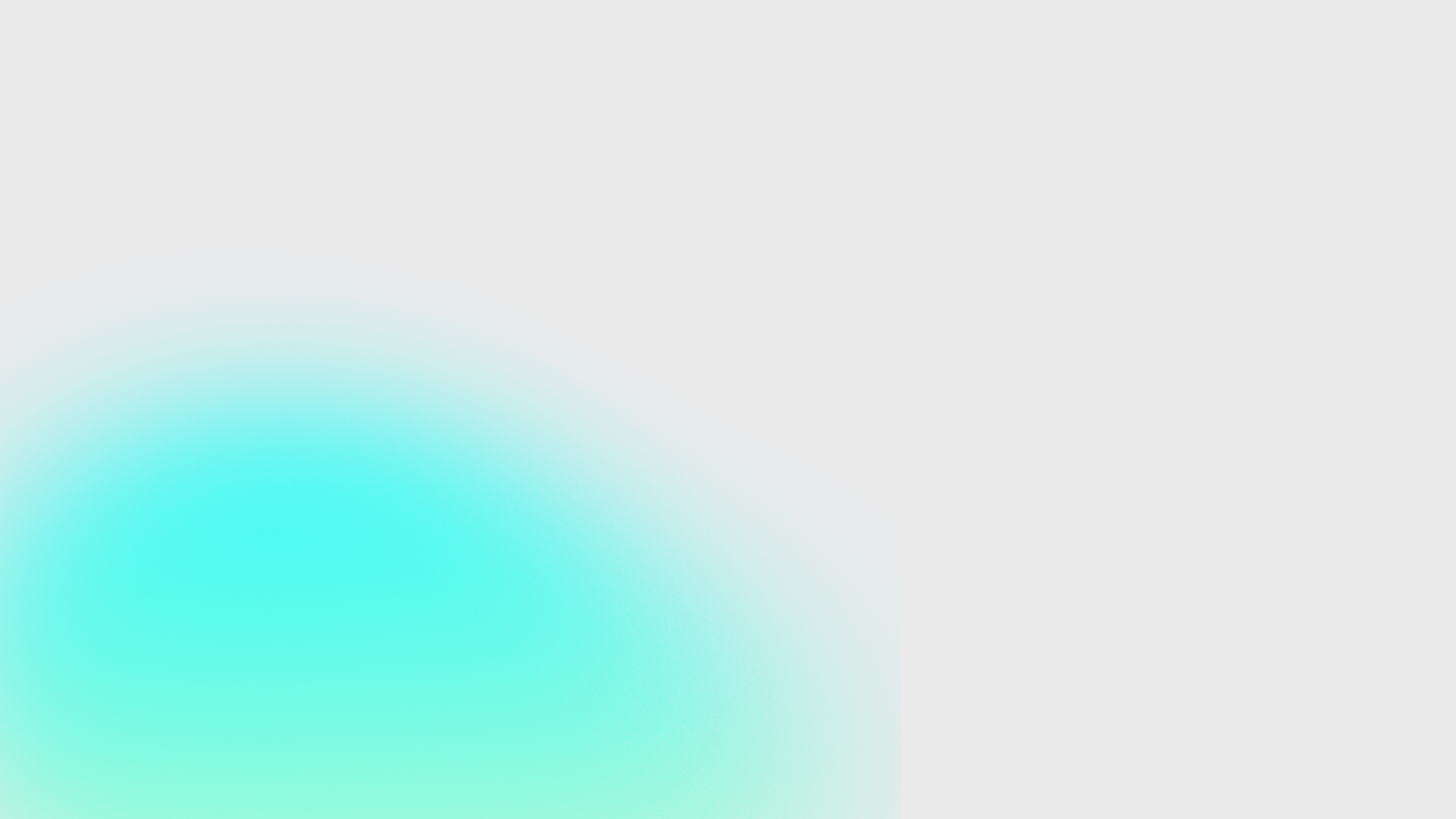
Contact us
Interested in working together? Fill out some info and we will be in touch shortly. We can’t wait to hear from you!